Think of the candidate gene as a targeted mission. Here, scientists focus on a few selected genes suspected of being involved in a particular disease. They already have a short list of genes to investigate, such as having a handful of suspects in a lineup. It’s like walking into a library with a specific book title in mind.
On the other hand, GWAS (Genome-Wide Association Studies) is a large-scale operation. Scientists embark on genome-wide searches, searching entire genetic libraries for clues. It seems they are searching every nook, every book on every shelf in search of the elusive genetic variation associated with a particular disease. This method does not require prior knowledge of which genes may be involved; This is a wide-net approach.
The candidate gene approach zooms in on a few genes previously suspected, while GWAS casts a wide net across the entire genome, hoping to stumble upon genetic mysteries hidden within. Both approaches are essential for unraveling the mysteries of our genetic code and its role in disease.
What is the Candidate Gene Approach?
The candidate gene approach is a method used in genetic research to investigate the genetic factors contributing to a specific trait or disease. It involves selecting a limited number of genes, often based on prior knowledge or hypotheses, and conducting detailed analyses to understand their potential association with the trait or disease of interest.
Key characteristics of the candidate gene approach include:
- Gene Selection: In this approach, researchers choose a small set of genes that they suspect may play a role in the trait or disease under investigation. These genes are selected based on existing knowledge of their biological functions or their potential relevance to the condition.
- Focused Analysis: Researchers then conduct focused and in-depth analyses of these selected genes. This may involve examining genetic variations (mutations, polymorphisms), gene expression levels, and protein function to identify associations with the trait or disease.
- Prior Knowledge: The candidate gene approach relies on prior knowledge or educated hypotheses about the genes that are likely to be involved. Researchers typically have a specific gene or group of genes in mind when they begin their investigations.
- Advantages: This approach offers the advantage of specificity and focus. It can be particularly effective when there is strong evidence linking the selected genes to the trait or disease, making it a targeted and efficient way to study genetic associations.
- Limitations: However, the candidate gene approach has limitations, as it may overlook other genes that could also be contributing to the trait or disease. It may not capture the full complexity of genetic factors involved, especially when multiple genes and interactions are at play.
The candidate gene approach is valuable when there is prior knowledge or a strong hypothesis about the genes involved in a particular condition. It has been successfully applied in various genetic studies, particularly when investigating well-characterized genetic disorders or traits.
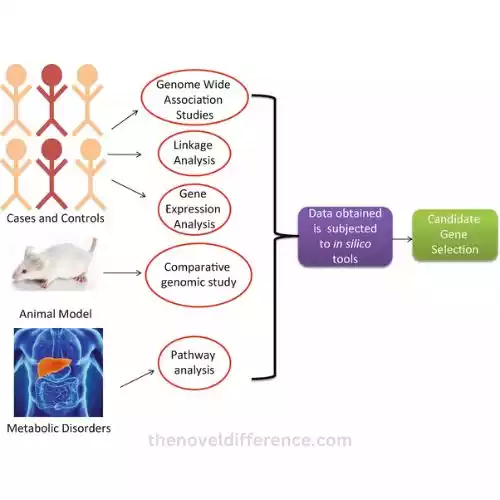
Why are candidate genes important?
Candidate genes are important in genetic research and the study of complex traits and diseases for several reasons:
- Focused Investigation: Candidate genes allow researchers to concentrate their efforts and resources on a limited number of genes that are highly likely to be involved in a specific trait or disease. This focused approach can be more efficient and cost-effective than genome-wide analyses, especially when there is substantial prior knowledge or strong hypotheses about the genes in question.
- Biological Relevance: Candidate genes are selected based on existing knowledge of their biological functions, pathways, or associations with the trait or disease. This prior knowledge makes it more likely that the selected genes have a direct role in the condition under study.
- Hypothesis Testing: The candidate gene approach enables researchers to test specific hypotheses or theories regarding the genetic basis of a trait or disease. It allows for targeted testing of associations between known genetic variants in these genes and the condition of interest.
- Clinical Relevance: When candidate genes are confirmed to be associated with a disease, they can have immediate clinical relevance. This knowledge may lead to the development of diagnostic tests, targeted therapies, and personalized treatment options.
- Reproducibility: Candidate gene studies with strong prior hypotheses are often more reproducible, as the findings can be validated by other researchers. This contributes to the robustness of scientific knowledge.
- Early Insights: Candidate gene studies can provide early insights into the genetic mechanisms underlying a trait or disease, offering starting points for further research and investigations.
- Focused Therapeutic Targets: When candidate genes are identified as playing a role in a disease, they can become valuable targets for the development of new drugs or therapies aimed at modulating their activity.
- Genetic Counseling: In clinical genetics and genetic counseling, knowledge of candidate genes can help provide patients and families with a better understanding of their genetic risks, prognosis, and potential preventive measures.
However, it’s important to note that the candidate gene approach also has limitations. It may miss novel genes or genetic factors not previously associated with the condition, and it might not capture the full complexity of genetic contributions, especially in the case of complex traits.
As a result, the candidate gene approach is often complemented by genome-wide association studies (GWAS) and other hypothesis-free methods to provide a more comprehensive view of genetic factors.
Why did candidate gene studies fail?
Candidate gene studies have faced challenges and limitations that have led to their shortcomings in certain cases. Some of the reasons why candidate gene studies may fail or produce inconclusive results include:
- Complex Nature of Traits: Many human traits and diseases are highly complex and are influenced by multiple genes, each with a small effect. Candidate gene studies often focus on single genes or a limited number of genes, which may not capture the full genetic complexity of the trait.
- Publication Bias: Positive results (i.e., significant associations between candidate genes and traits) are more likely to be published, while negative results (lack of association) are often not reported. This can lead to an overrepresentation of false positive findings in the scientific literature.
- Small Sample Sizes: Some candidate gene studies may have insufficient sample sizes to detect subtle genetic effects. Inadequate statistical power can result in false-negative findings.
- Multiple Testing Issues: Candidate gene studies frequently involve testing multiple genetic variants within a single gene. Without proper statistical correction for multiple comparisons, there is an increased risk of obtaining false-positive results.
- Genetic Heterogeneity: Genetic contributions to traits and diseases can vary among different populations or ethnic groups. Candidate gene studies may not account for this heterogeneity, leading to inconsistent findings.
- Incomplete Genetic Understanding: In some cases, prior knowledge about candidate genes may be based on incomplete or outdated information. Genes initially thought to be relevant may not play a significant role in the condition under study.
- Gene-Gene and Gene-Environment Interactions: Candidate gene studies often focus on individual genes and may not capture interactions between genes or gene-environment interactions, which can be crucial for understanding genetic contributions to complex traits.
- Publication Pressure: Researchers and journals may have a bias toward publishing positive results, potentially leading to the underreporting of negative or inconclusive findings.
- Lack of Replication: Some candidate gene studies have reported positive associations, but subsequent attempts to replicate these findings in independent studies have failed. Replication is a crucial step in validating genetic associations.
As a result of these challenges, candidate gene studies are sometimes viewed with caution, and their findings may need to be independently validated through larger, more comprehensive approaches, such as genome-wide association studies (GWAS) or other hypothesis-free methods. These limitations have contributed to the shift toward more unbiased and comprehensive genetic research approaches in recent years.
What is the GWAS (Genome-Wide Association Studies)?
Genome-wide association Studies (GWAS) is a comprehensive and hypothesis-free research approach used to identify genetic variations associated with specific traits, diseases, or conditions. GWAS analyzes the entire genome, examining genetic markers (such as single nucleotide polymorphisms or SNPs) across a broad spectrum of genes to pinpoint variations that may be linked to the trait or disease in question.
Key characteristics of GWAS include:
- Unbiased Genome-Wide Analysis: Unlike the candidate gene approach, GWAS does not rely on prior knowledge or hypotheses about specific genes. Instead, it examines thousands to millions of genetic markers distributed across the entire genome, providing a comprehensive view of the genetic landscape.
- Large-Scale Data Collection: GWAS involves collecting genetic data from a large and diverse group of individuals with and without the trait or disease of interest. This extensive dataset is crucial for identifying statistically significant associations.
- Statistical Analysis: The data generated from GWAS is subject to advanced statistical analyses to detect genetic variants that are more common in individuals with the trait or disease. These variants are known as risk alleles or associated markers.
- Discovery of Novel Associations: GWAS has the potential to uncover previously unknown genetic variations that contribute to a particular condition. This makes it a powerful tool for exploring the genetic underpinnings of complex traits and common diseases.
- Polygenic Nature: Many traits and diseases are influenced by multiple genetic variants, each with a small effect. GWAS identifies these polygenic associations, helping scientists understand the combined genetic factors contributing to a trait or disease.
- Genetic Risk Scores: The results of GWAS can be used to calculate genetic risk scores, which estimate an individual’s genetic predisposition to a particular condition. This information has applications in personalized medicine and risk assessment.
- Applications: GWAS has been applied to investigate a wide range of conditions, from common diseases like diabetes and cardiovascular disorders to complex traits such as height and cognitive abilities. It has also contributed to the identification of potential therapeutic targets.
GWAS is a powerful approach for exploring the genetic basis of complex traits and diseases. While it doesn’t provide immediate insights into the biological functions of the identified genetic markers, it serves as a crucial starting point for further research into the underlying mechanisms and potential interventions.
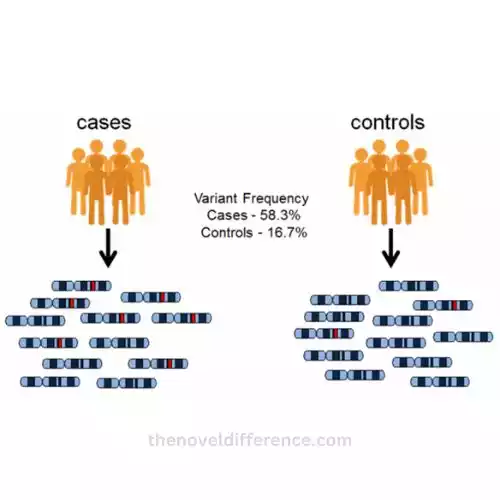
What is the basic principle of GWAS?
The basic principle of a Genome-Wide Association Study (GWAS) is to systematically scan the entire genome to identify genetic variations that are statistically associated with a specific trait, disease, or characteristic.
The fundamental steps and principles of a GWAS are as follows:
- Genome-Wide Analysis: GWAS examines a vast number of genetic markers, typically single nucleotide polymorphisms (SNPs), spread across the entire genome. SNPs are variations in a single DNA base pair and serve as markers for genetic diversity.
- Case-Control Design: A GWAS typically involves two groups of individuals: cases (individuals with the trait or disease of interest) and controls (individuals without the trait or disease). This design allows for comparisons to identify genetic variations that are more common in cases.
- Genotyping: In a GWAS, the genetic markers (SNPs) are genotyped, which means determining the specific genetic variants an individual carries. This is done for both cases and controls.
- Statistical Analysis: Advanced statistical analyses are applied to the genotyping data to identify SNPs that are associated with the trait or disease. These analyses assess whether specific SNPs are more or less common in cases compared to controls.
- Significance Threshold: A significance threshold or p-value cutoff is set to determine which associations are statistically significant. SNPs that surpass this threshold are considered associated with the trait or disease.
- Replication and Validation: Positive findings from a GWAS should ideally be replicated in independent study populations to confirm the associations. Replication helps ensure that the observed genetic associations are not due to chance.
- Biological Interpretation: Once significant associations are identified, researchers aim to understand the biological mechanisms underlying these genetic variations and how they may contribute to the trait or disease.
The key principle behind a GWAS is that it is a hypothesis-free approach, meaning it does not rely on prior knowledge or hypotheses about specific genes. Instead, it casts a wide net across the entire genome to uncover genetic factors that may be linked to a trait or disease.
This unbiased and comprehensive approach has led to the discovery of numerous genetic associations and has been instrumental in advancing our understanding of the genetic basis of complex traits and common diseases.
Why are SNPs used in GWAS?
Single Nucleotide Polymorphisms (SNPs) are commonly used in Genome-Wide Association Studies (GWAS) for several compelling reasons:
- Common and Abundant: SNPs are the most common type of genetic variation in the human genome. They occur frequently, with an estimated average of one SNP per 300 base pairs. This abundance allows for a high density of genetic markers to be analyzed in a GWAS.
- Stable and Inherited: SNPs are relatively stable and can be reliably inherited from one generation to the next. This makes them suitable for tracking genetic variations associated with traits and diseases.
- Biological Relevance: Some SNPs can have functional consequences, influencing gene expression, protein structure, or other biological processes. These functional SNPs are of particular interest in understanding the genetic basis of traits and diseases.
- Ease of Genotyping: Genotyping SNPs is a well-established and cost-effective process, making it feasible to analyze a large number of genetic markers in a GWAS.
- Linkage Disequilibrium: SNPs are often in linkage disequilibrium (LD) with other nearby genetic variants. This means that the presence of one SNP can be indicative of the presence of other nearby SNPs, allowing for the identification of associations with multiple genetic variations.
- Statistical Power: Analyzing a large number of SNPs in a GWAS enhances its statistical power to detect associations with traits or diseases, especially when those associations are driven by multiple genetic factors with small effects.
- Population Differences: SNPs can vary among different populations and ethnic groups, providing insights into how genetic associations with traits or diseases may differ across populations.
- Biological Interpretation: SNPs that are associated with a trait or disease can potentially lead to insights into the underlying biological mechanisms that contribute to the condition.
SNPs serve as practical genetic markers for GWAS, allowing for the exploration of the genetic architecture of complex traits and common diseases in a systematic and unbiased manner. Their abundance, stability, and biological relevance make them valuable tools for identifying genetic associations.
Candidate Gene and GWAS in the key comparison chart
Here’s a key comparison chart summarizing the main differences between Candidate Gene Studies and Genome-Wide Association Studies (GWAS):
Characteristic | Candidate Gene Studies | GWAS (Genome-Wide Association Studies) |
---|---|---|
Focus | The targeted approach investigates a limited number of pre-specified genes | Unbiased and comprehensive, explores the entire genome |
Gene Selection | Genes are selected based on prior knowledge or hypotheses about their relevance | No prior gene selection, all genetic markers are analyzed |
Hypothesis-Driven | Hypothesis-driven relies on prior knowledge or specific gene hypotheses | Hypothesis-free aims to discover novel genetic associations |
Number of Genes Analyzed | Analyzes a small number of genes, often less than a dozen | Analyzes a vast number of genetic markers (e.g., millions of SNPs) |
Scope | Narrow in scope, focuses on known genes of interest | Broad in scope, explores the entire genome for associations |
Replication Requirement | Often requires independent replication to validate findings | Replication is essential for validating identified associations |
Complex Traits | May be suitable for simple or well-understood traits and diseases | Suited for complex traits and common diseases with multiple genetic factors |
Statistical Correction | Fewer multiple testing corrections are required due to the smaller gene set | Requires stringent multiple testing corrections due to the larger number of markers |
Advantages | Specificity and focus on known genes, potential for well-informed hypotheses | Comprehensive and unbiased, capable of identifying novel genetic associations |
Limitations | May miss unknown or novel genetic factors, limited to known genes | Potential for false positives, complex statistical analyses, higher resource requirements |
Clinical Applications | Suitable for targeted genetic testing and diagnosis based on known genes | Provides broad genetic insights with potential for personalized medicine |
Historical Use | The common approach in earlier genetic research | Modern approach in contemporary genetics research |
This chart provides a quick overview of the key differences between Candidate Gene Studies and GWAS, highlighting their focus, approach, number of genes analyzed, replication requirements, suitability for complex traits, and more. These methods serve different purposes in genetic research and can be complementary in gaining a comprehensive understanding of genetic associations.
Similarities of Candidate Gene and GWAS (writ unique word)
Although Candidate Gene Studies and Genome-Wide Association Studies (GWAS) have distinct approaches and methodologies, they share some commonalities in their role and impact within the field of genetic research.
Some of these shared aspects include:
- Genetic Associations: Both Candidate Gene Studies and GWAS aim to identify genetic associations with specific traits, diseases, or characteristics. They are instrumental in revealing how genetic variations contribute to these outcomes.
- Contributions to Genetic Knowledge: Both approaches contribute to the broader understanding of the genetic basis of traits and diseases. Candidate Gene Studies may uncover specific gene-trait relationships, while GWAS can reveal novel genetic associations.
- Relevance to Personalized Medicine: Findings from both types of studies can have implications for personalized medicine and clinical applications. They may inform risk assessments, diagnostic tests, and treatment strategies tailored to an individual’s genetic profile.
- Replication and Validation: In both cases, replication and validation of results are critical steps to ensure the reliability and robustness of genetic associations. Consistent findings across independent study populations increase confidence in the identified associations.
- Advancements in Disease Understanding: Both methods contribute to advancements in understanding the genetic underpinnings of various diseases and conditions, which can lead to the development of targeted therapies and interventions.
- Statistical Analysis: Both Candidate Gene Studies and GWAS involve advanced statistical analyses to assess the strength of associations and control for potential confounding factors. This shared statistical rigor ensures the validity of findings.
- Multifactorial Traits: Both methods can be applied to study traits and diseases with complex genetic contributions, including those influenced by multiple genetic factors, environmental factors, and gene-environment interactions.
- Collaboration and Data Sharing: Researchers often collaborate and share data across studies, whether they are conducting Candidate Gene Studies or GWAS. This collaborative approach helps validate findings and accelerates scientific progress.
Despite their differences, Candidate Gene Studies and GWAS ultimately work together to expand our understanding of genetics and its role in shaping human health, and they continue to be vital tools in the realm of genetic research.
Conclusion
Studying genetic factors in human traits and diseases is of great importance. Genetic research helps us understand the underlying mechanisms that contribute to the development and manifestation of traits and diseases.
By investigating genetic variations, scientists can identify potential biomarkers, therapeutic targets, and personalized treatment approaches. Genetic Studies Contribute to our Knowledge of Disease Risk Factors, Heritability Estimates, and Gene-Environment Interactions, Leading to Improved Disease Prevention and Management Strategies.